Save 99% power for audio processing thanks to a new analog approach
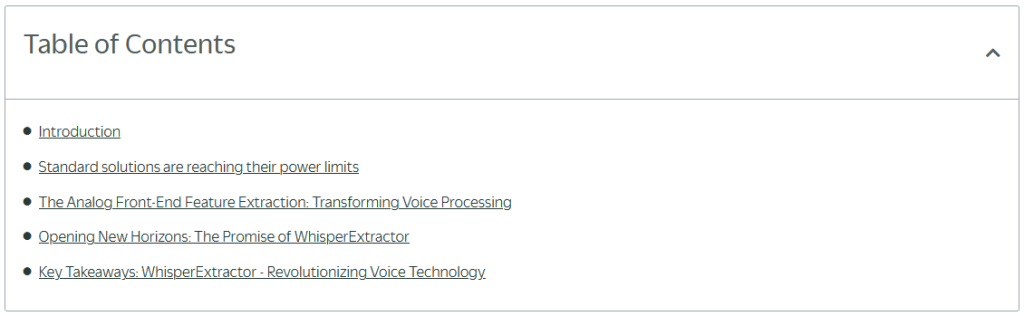
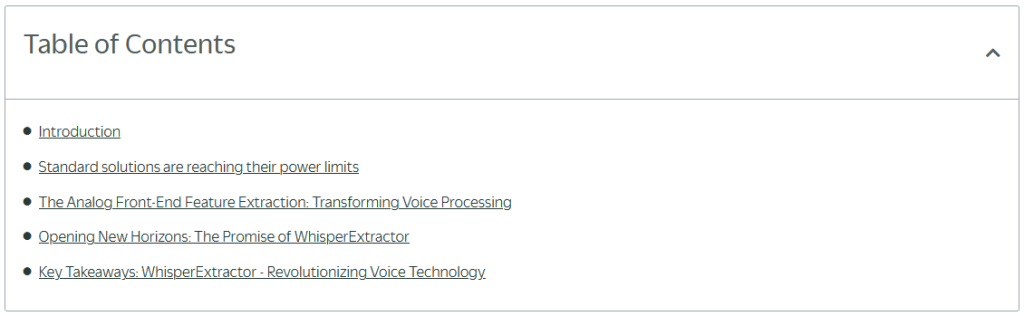
Dolphin Design is tackling a major challenge in making voice user interface (VUI) truly ultra-low-power to enhance end-user experience.
In our rapidly evolving human-to-machine interface landscape, voice-user interaction has become an integral part of our daily lives, transforming the way we engage with technology. Devices we can talk to (voice command) are now a common part of our homes, workplaces, and even our pockets, announcing a new era of convenience and efficiency.
However, with this surge in voice interaction adoption comes a significant challenge – efficient acquisition, pre-processing (feature extraction), and Artificial intelligence (AI) processing (inference).
Conventional methods of audio acquisition and feature extraction have proven to be energy-intensive, obstructing the implementation of really low-power always-on voice listening capabilities, particularly in resource-constrained devices like smart glasses, hearables, and remote controls. These traditional signal acquisition and processing approaches involve analog-to-digital signal conversion and subsequent computational operations, demanding a substantial power budget.
You can find out more by downloading the white paper.