
Revolutionary ultra-low power SoC architectures
CPU-less data management subsystems enablement for ultimate energy efficiency

Subsystem architectures are critical to energy savings
Designers usually build their SoC based on pre-designed & pre-verified subsystem architectures.
The architecture choice is extremely important to maximize energy efficiency. We have experienced more than 100x improvement in energy efficiency thanks to novel subsystem architectures. Innovating in this area is definitely the right path to push back SoC energy efficiency limits.

With MAESTRO, speed-up your SoC design with a configurable Power Controller RTL IP
With the growing chip complexity observed in many market segments, in addition to a need for longer battery life, SoC design teams are forced to adopt advanced power management techniques to improve the energy efficiency of their devices. Recent process nodes offer a high level of SoC integration with RF connectivity, logic MCU, non-volatile memory, AI/ML processor, and analog blocks on the same die.
Designing a custom logic to control the devices involved in power management requires an in-depth expertise and drastically increases the design and verification cycle time.
We created MAESTRO, a unique Power Controller RTL IP which is accompanied by PowerStudio compiler to speed-up the design of a highly scalable power controller that features breakthrough capabilities compared to traditional HW or SW PMU design methodologies.
CPU-less data management subsystems are the future for ultimate energy efficiency gains, discover CHAMELEON

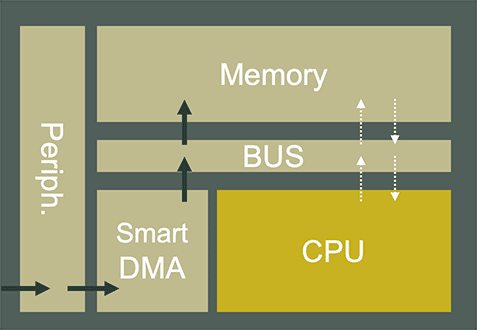
CPU-centric subsystem are limited in energy efficiency
In CPU-centric architecture (the most commonly used) the CPU is involved in every data event on peripherals. In deep sleep mode, the CPU context needs to be restored for each data event, leading to significant latency and power overhead.
Moreover, while the CPU is busy treating the data, it cannot perform other tasks, leading to severe performance limitations.
CPU-less data management system architectures do not suffer from the limitations of the CPU-centric architecture.
The data can be autonomously stored and treated through a smart DMA without involving the CPU, leading to significant power & latency savings. Moreover, with an improved bus & memory architecture, the CPU can perform other tasks and access the memory in parallel, leading to drastic performance improvements.
We have developed CHAMELEON, a unique subsystem approach based on a CPU-less data management system technology.
Tiny RAPTOR, a Power-efficient Neural Processing IP Platform specialized in sound and vision
A specialized neural processor that implements up to 128 processing elements and the necessary control and arithmetic logic to execute Machine Learning Algorithms of predictive models.
Tiny RAPTOR is a fully programmable accelerator designed to execute deep neural networks (DNN) in an energy-efficient way. It reduces the inference time needed to run Machine Learning (ML) Neural Networks (NN). Tiny RAPTOR fits particularly well within any MCU subsystem, in particular with our MCU subsystem CHAMELEON.
Parallel & versatile processing for greater Energy-Efficiency & SoC flexibility, meet PANTHER
PANTHER is a scalable multi-core Digital Signal Processor IP Platform capable to efficiently run typical signal processing tasks as well as Artificial Intelligence and Machine Learning algorithms.
PANTHER relies on a popular RISC-V instruction set and leverages a rich software ecosystem. Configurable from 4 to 16 parallel cores that rely on an advanced memory interconnect, PANTHER combines ultimate throughput efficiency with optimal silicon area to fit ideally with each SoC requirement.
Software execution on its parallel architecture is streamlined through the delivery of dedicated libraries together with a complete software development kit.

Need to discuss about your project?
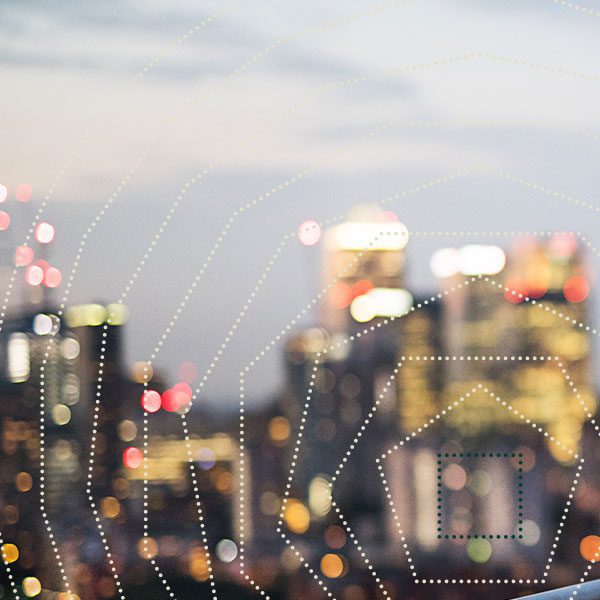